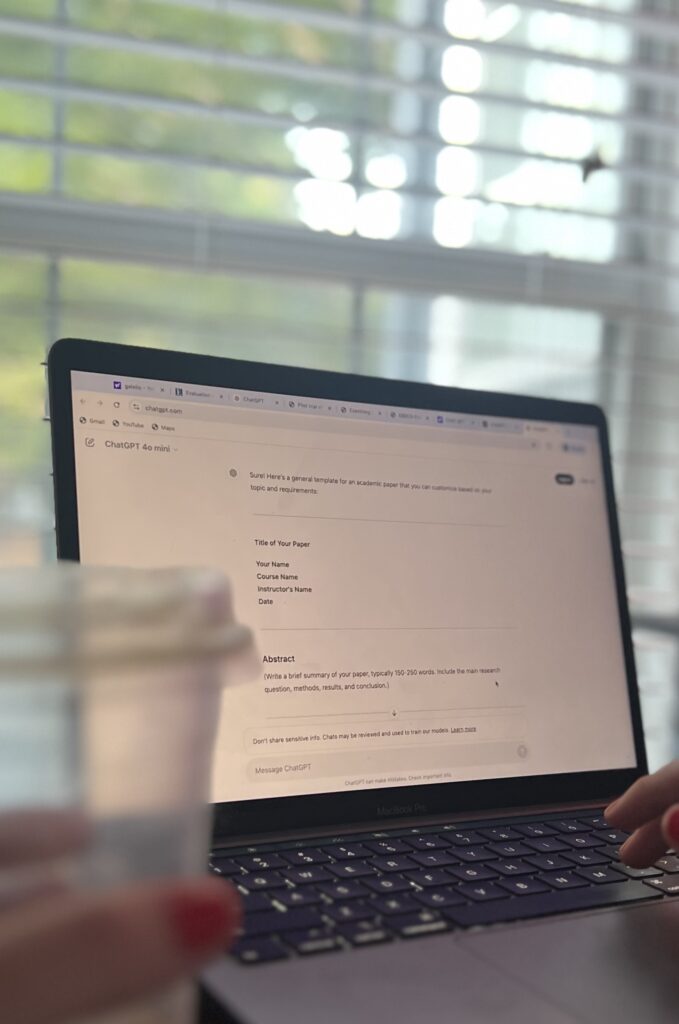
Artificial intelligence has captured public attention, sparking confusion and excitement; while AI’s potential is often seen as limitless, public understanding remains limited— making AI literacy a potential advantage in navigating a time of technology.
In a society where ai continues to weave itself into daily life —from automating tasks to enhancing decision-making— many are left wondering how to harness its potential effectively and easily. While ai holds great promise, users should remember that these models are not “magic genies that can output whatever”, but rather customizable assistants that work best when given clear instructions alongside accurate data. This process of instruction is known as prompt engineering, which can greatly increase the accuracy of the next predicted token making answers and interactions more tailored to users needs.
“But then how can you use them in real life? I mean you can use them… for everything” -donnie
Getting started: understanding AI Models and Their Practical use
Before users can effectively maneuver these models for practical use, it’s essential to understand the basic principles of large language models (LLMs) and artificial intelligence before integration. These models are powered by algorithms trained on vast datasets, to recognize patterns and predict outcomes . However AI doesn’t have a built-in responsibility to the truth, it generates responses only on the data it has processed.
Once the user’s desired data is input, they can pose different questions referred to as “prompts”; when structured properly, prompts could yield more relevant information fitting the users parameters. For tasks planning purposes such as, emails, organizing documents, time management, project planning, prompt engineering can be very useful as the parameters are clearly defined and straightforward often producing “near human like output”. While this process offers users a lot, this alone won’t solve higher order thinking tasks in a one shot prompt attempt.
Donnie believes that this points to a larger issue, which is the challenges data scarcity poses due to the high cost, time, and energy involved in collecting and fine-tuning data. Due to these models lacking an inherent understanding of or responsibility to the truth, leads to responses that might sound impressive but aren’t always accurate.
As Tyson explains, AI models can make mistakes, often “hallucinating” by generating information that is either irrelevant or completely fabricated. Despite this, they will still produce a response, regardless of its accuracy, which highlights the need for critical oversight in their use.
“Um, you know, I, I think that, uh, that kind of stuff is really sobering.” – Tyson
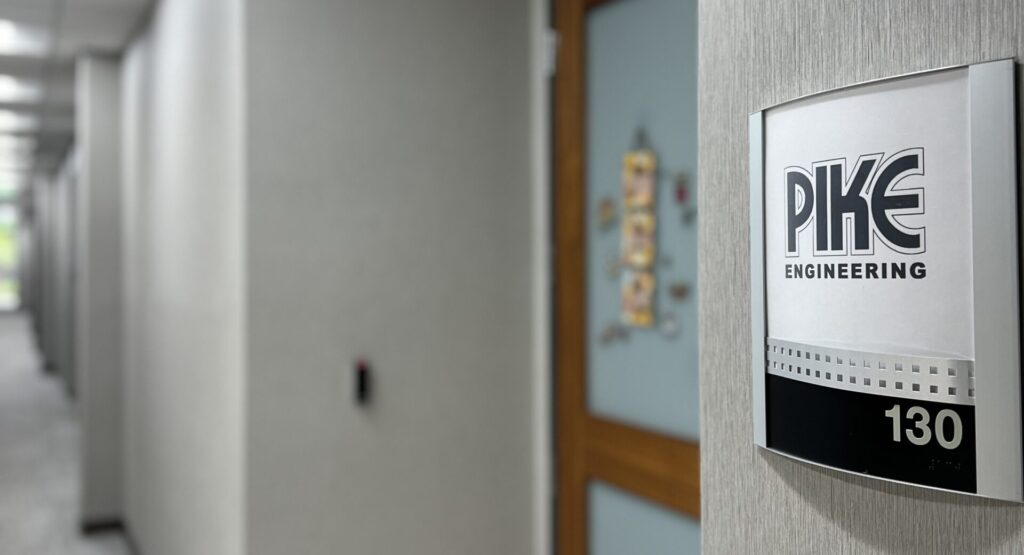
Prompting techniques for maximizing applicable output
Multi-shot prompting offers consistency and clarity, useful for nuanced tasks or structured problem-solving. Using examples, the model can better capture the desired tone or address gaps in understanding. It’s ideal for writing, coding, or tasks with defined steps, reducing variability and producing more tailored responses.
Multi-shot prompting uses multiple examples to guide the model toward responses with a specific format, tone, or depth. Starting with a contextual instruction like, “Please respond to each question in a complete sentence,” the prompt includes examples with a question-answer or task-output pair, showing the desired response style. This format reinforces the intended style for consistent answers.
Chain-of-thought prompting sequence questions to guide the model through logical reasoning. For example, when analyzing a scientific experiment, a chain-of-thought prompt may explore steps like hypothesis, variables, and outcomes. This method supports complex decision-making and multi-step analysis by structuring the reasoning process. This process improves the model’s reasoning ability, guiding it through multi-step tasks, as it now has a “chain-of-thought” process to reference or for the user to reference.
Chain-of-thought prompting improves the model’s reasoning ability, guiding it through multi-step tasks. Structured prompts help the model focus and adapt to specific instructions, enhancing accuracy and relevance for tasks requiring sequential reasoning.
Integration and application
Combining multi-shot and chain-of-thought prompting creates a structured approach for complex projects. Multi-shot prompting establishes a foundational tone, while chain-of-thought guides logical analysis. This iterative approach is ideal for tasks similar to drafting reports, allowing consistent voice and depth in sections needing detailed explanation. As one expert in the AI consulting field noted, “Prompt engineering is akin to interviewing someone,” emphasizing the guidance structured prompts provide.
This workflow can also support educational tasks, like teaching division, by using multi-shot prompting to introduce familiar operations and then using a chain-of-thought prompting technique or inquiry to break down new concepts. This approach builds foundational knowledge progressively, aligning outputs with specific learning goals. This method adapts to the learner’s needs, progressively building knowledge in a structured path toward mastery while adapting its approach based on your needs and own discovery.
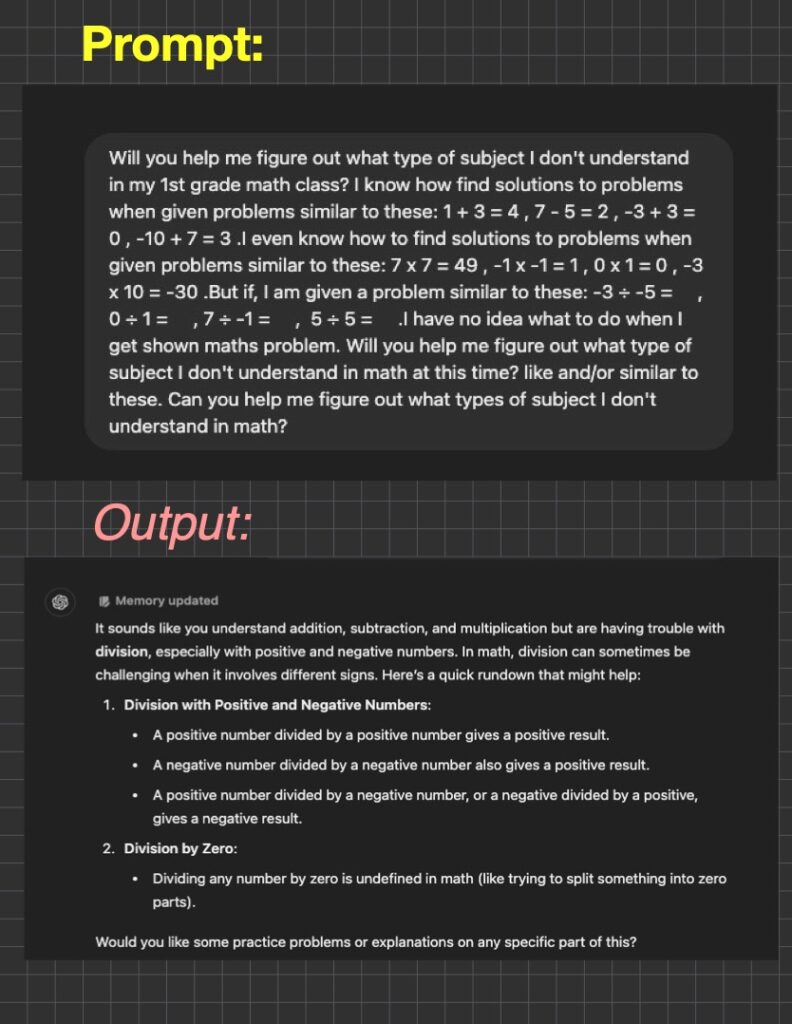
Considered Elements For Prompting Purpose
- Relevant examples
- Example Diversity
- Instruction Placement
- Clarity and concision
Application of these elements can vary between use cases but the purpose of them remains similar. These elements aim to create layered parameters making a more clear understanding for the model to base its responses off of.
Iterative workflows
In educational contexts, an iterative workflow could begin with multi-shot prompting to set a familiar context, followed by chain-of-thought prompting to explore new concepts step-by-step or for you to interject your approach as opposed to using concepts from these steps. This method adapts to the learner’s needs, progressively building knowledge in a structured path toward mastery while adapting its approach based on your needs and own discovery.
An iterative workflow for learning, like division, starts with multi-shot prompting to set context and follows with a chain-of-thought response to break down each step. By alternating these techniques, learners build connections between familiar and new concepts, gaining a comprehensive understanding through a structured path.
Iterative workflows offer a structured yet adaptable framework for tackling complex tasks by breaking them into manageable steps. Combining multi-shot and chain-of-thought prompting, users build knowledge progressively, connecting new ideas to familiar concepts. This flexibility supports both learning and problem-solving, fostering comprehension through a customized path to understanding and exploration.
Benjamin Adams, a senior at UGA, walks through a use case example with OpenAI’s GPT 4-o to demonstrate how users can use multi-shot prompting to directly apply their current understanding of a topic to an area of uncertainty within the same topic; he does this by highlighting techniques he learned from his outside research, continual use, and application gained from his enterprise story, titled, AI Literacy: Not Negating The Basics.
Output from OpenAI’s ChatGPT-4o model for use case example
‘Photosynthesis changes the way flowers grow by providing them with the energy needed for development and reproduction. Through this process, plants convert sunlight, water, and carbon dioxide into glucose and oxygen. The glucose acts as a source of energy and a building block for growth, enabling flowers to mature and produce blooms effectively. Additionally, photosynthesis supports the creation of strong stems, healthy leaves, and vibrant petals by facilitating nutrient absorption and cell formation.’
Show Comments (0)